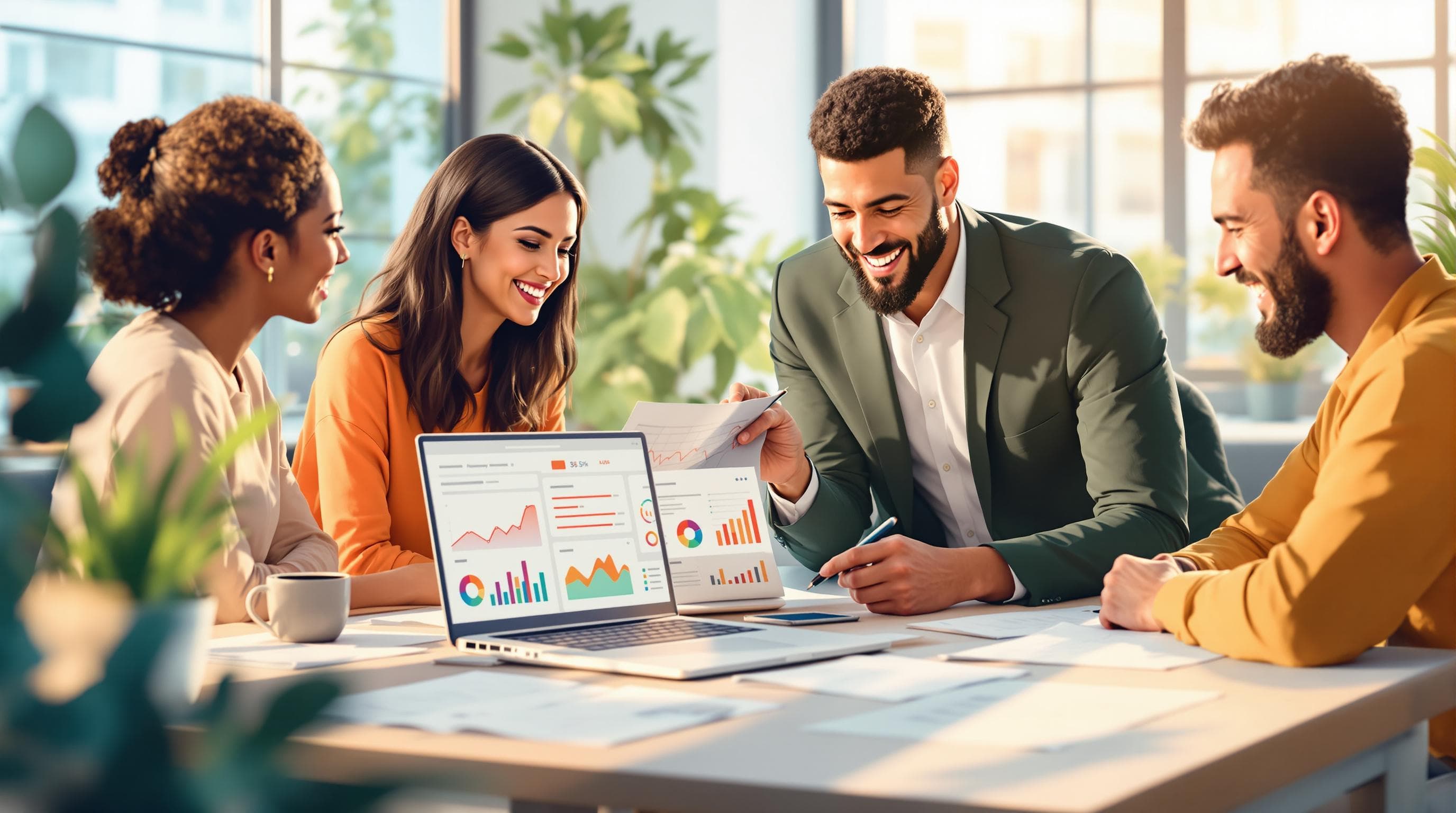
Common Customer Feedback Analysis Challenges Solved
Analyzing customer feedback can be tough, but AI tools are making it easier. Businesses face three big challenges:
- Too much unstructured data: Social media, surveys, and emails can overwhelm manual methods.
- Sentiment detection issues: Misreading sarcasm, mixed emotions, or cultural context leads to errors.
- Handling multiple channels: Feedback from different platforms often gets lost or misused.
AI solves these problems by automating analysis, improving sentiment accuracy, and organizing feedback efficiently. Tools like SentiSum and Zonka Feedback help businesses turn feedback into actionable insights for better decisions and customer experiences.
Ready to dive deeper? Let’s explore how AI transforms feedback analysis.
Master Customer Feedback Analysis with AI
Main Challenges in Analyzing Customer Feedback
Analyzing customer feedback has grown more complicated as businesses gather data from a variety of platforms. Here’s a closer look at the key hurdles organizations encounter when trying to make sense of customer feedback.
Tackling Unstructured Feedback
Free-text responses, social media comments, and other unstructured feedback hold a wealth of insights, but extracting these insights systematically can be tough without the right tools. Manual analysis is time-consuming and often prone to mistakes, making automation a practical necessity. While thematic analysis can help identify recurring patterns, many companies find it difficult to process this type of feedback efficiently .
Even when feedback is categorized, understanding the emotional tone behind it presents its own set of difficulties.
Navigating Sentiment Detection Challenges
Interpreting customer sentiment isn’t always straightforward. Language nuances like sarcasm, mixed feelings, or unclear phrasing can easily lead to misinterpretations of customer intent .
Here’s a breakdown of common sentiment detection hurdles:
Challenge | Impact & Common Pitfall |
---|---|
Sarcasm Detection | Misreading sarcastic remarks as literal statements |
Mixed Emotions | Reducing feedback to a single emotion, ignoring its complexity |
Cultural Context | Misjudging feedback by applying one-size-fits-all sentiment rules |
Vague Language | Guessing intent when feedback lacks clarity |
Handling High Volumes of Feedback
When feedback comes in large quantities, managing it effectively requires systems that can handle diverse sources, maintain consistency, and scale as needed - all while ensuring data quality. AI and machine learning have made it easier to spot trends and organize feedback, even when dealing with massive datasets.
Real-time analysis introduces another layer of difficulty, as businesses must deliver accurate and actionable insights quickly. Key priorities include:
- Processing data from multiple channels at once
- Ensuring consistent analysis across various feedback formats
- Scaling systems to accommodate growing feedback volumes
- Maintaining high standards for data quality and validation
These challenges emphasize the importance of advanced tools to process feedback both efficiently and accurately. The next section will dive into how AI-driven solutions can help businesses address these obstacles .
sbb-itb-bca9945
AI Solutions for Feedback Analysis Problems
AI tools are changing the way businesses handle, interpret, and act on customer feedback.
AI for Sentiment Analysis
With advanced NLP, AI can pick up on cultural differences, handle multiple languages, catch subtle emotional cues, and process large volumes of feedback in real-time.
Sentiment Analysis Feature | What It Does |
---|---|
Context Understanding | Detects cultural and situational differences |
Multi-language Support | Handles feedback in various languages |
Real-time Processing | Quickly analyzes thousands of entries |
Nuanced Detection | Captures subtle emotional cues |
By solving challenges in sentiment detection, AI turns feedback into a solid base for making informed decisions.
Organizing Feedback from Different Channels
AI simplifies managing feedback from multiple sources. These tools automatically sort unstructured feedback into themes, making it easier for teams to spot patterns and focus on priorities.
Ensuring Data Accuracy and Context
Accurate feedback analysis depends on clean data and proper context. AI enhances accuracy by detecting errors in real-time, cross-referencing data from various sources, and understanding industry-specific language and customer communication styles.
A great example is SentiSum, which processes feedback from all customer channels to provide a comprehensive view of customer sentiment . These AI advancements help businesses go beyond just collecting feedback, turning it into actionable insights to improve customer experiences.
Steps for Using AI in Feedback Analysis
Choosing the Right Tools
Pick AI tools that address your specific feedback challenges effectively.
Key Tool Features | Importance |
---|---|
Advanced Sentiment Analysis | Helps uncover emotional tones and contextual nuances in feedback |
Theme Customization | Lets you tailor analysis to fit your industry or business needs |
Data Security | Protects sensitive customer information |
Integration Capabilities | Ensures compatibility with systems like CRM platforms |
Tools like Painboard simplify the process by merging themes automatically, eliminating the need for manual adjustments. This makes the analysis process smoother and more aligned with business requirements.
Defining Clear Objectives for Analysis
To get the most out of AI-powered feedback tools, set well-defined, measurable goals. Focus on actionable outcomes, such as identifying common customer pain points or tracking improvements in specific service areas.
For example, platforms like Zonka Feedback allow businesses to identify recurring themes and directly link them to measurable KPIs like NPS or CES. Once goals are set, ensure your AI tools can handle increasing feedback volumes and integrate seamlessly into your existing processes.
Prioritizing Scalability and Integration
Choose cloud-based solutions that can grow with your business needs without sacrificing performance. Tools like SentiSum offer cloud infrastructure that scales easily as feedback volumes increase .
For smooth integration:
- Link AI tools to your current systems.
- Automate data validation processes.
- Train your team to turn insights into meaningful actions.
Conclusion: Turning Feedback into Business Insights
Many businesses face challenges when it comes to analyzing customer feedback. Issues like unstructured data, misreading sentiment, and overwhelming volumes make it hard to extract useful information. AI-powered tools are changing the game by transforming feedback into actionable insights. For example, Painboard tackles these challenges with features like automated theme detection and sentiment analysis.
Industry data highlights how AI-driven feedback tools provide more precise insights and quicker response times. A great example is Zonka Feedback, which uses AI to efficiently spot patterns and gaps in customer feedback .
These tools directly address the earlier challenges of handling unstructured data, improving sentiment accuracy, and scaling feedback analysis. Here's how they impact key business areas:
Business Area | AI-Powered Impact |
---|---|
Product Development | Identifies customer pain points and issues in real time |
Customer Service | Detects and resolves issues proactively |
Strategic Planning | Enables decisions based on data |
Quality Assurance | Monitors satisfaction automatically |
The key to success lies in selecting the right tools and using them wisely. Painboard’s centralized approach to managing feedback, combined with a strong focus on data security, demonstrates how businesses can scale analysis efforts while maintaining accuracy. By integrating AI into their strategy, companies can turn customer feedback into a powerful edge over competitors.