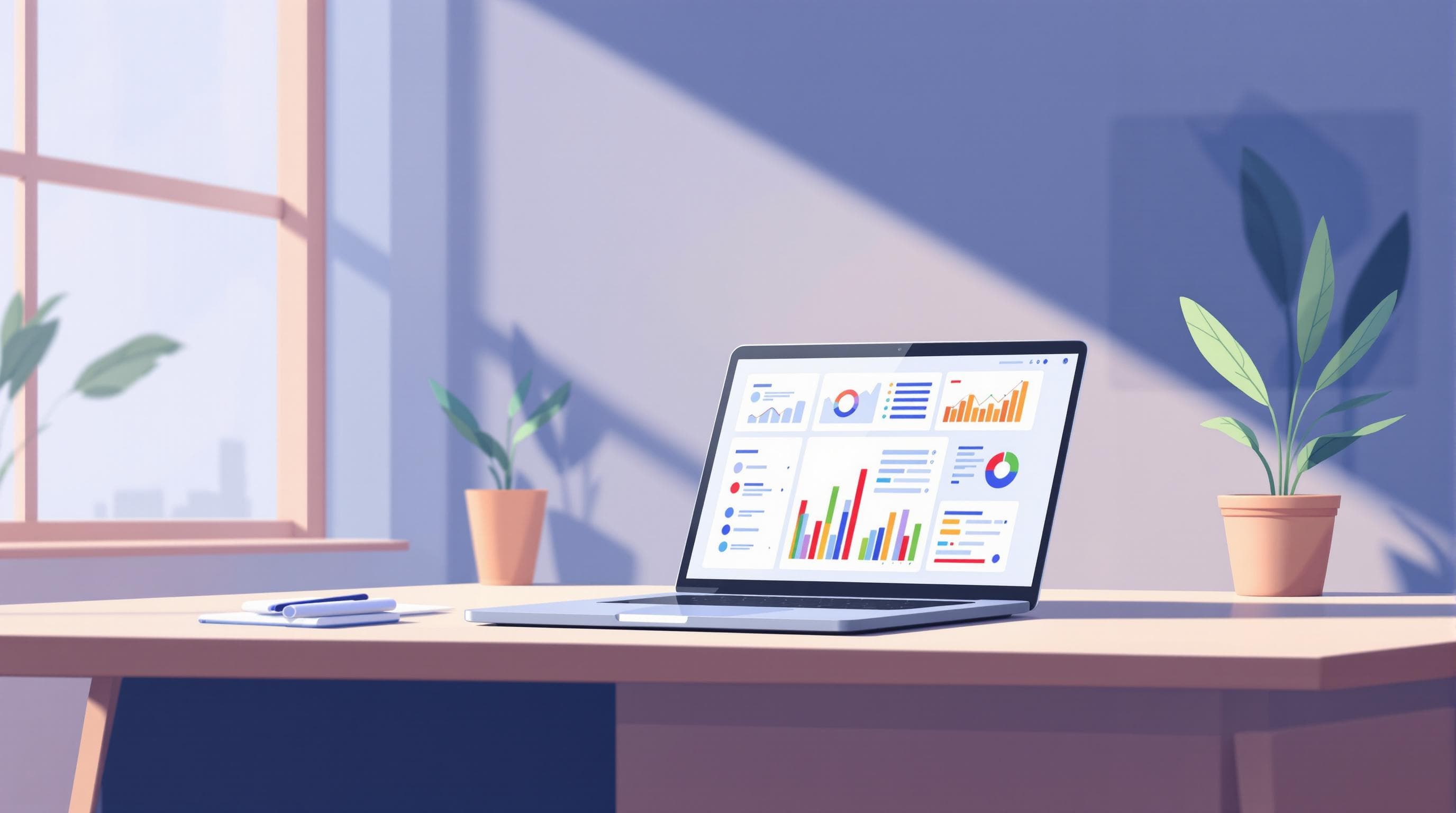
Scaling Customer Feedback Analysis: Key Considerations
Scaling customer feedback analysis is essential for businesses to handle growing data and improve decision-making. Here's what you need to know:
- Why It Matters: Only 22% of businesses feel confident in analyzing feedback effectively, despite its importance for growth.
- Key Challenges: Unstructured data, resource limitations, and data quality issues make scaling difficult.
- Solutions:
- AI Sentiment Analysis: Real-time insights, multi-language support, and trend detection.
- Data Visualization: Tools like clustering and trend mapping simplify feedback interpretation.
- Custom Workflows: Automate collection, categorization, and routing for efficiency.
- Data Quality: Ensure accuracy with validation, cleaning, and governance practices.
Customer Sentiment Analysis AI Explained
Using AI for Sentiment Analysis
AI-driven sentiment analysis has changed the way businesses handle and interpret customer feedback on a large scale.
Why Use AI for Sentiment Analysis?
AI sentiment analysis offers powerful advantages that address key shortcomings of traditional methods, such as the 22% confidence gap in manual analysis. According to The Boston Consulting Group, companies using advanced customer experience analytics saw 190% higher three-year revenue growth and 70% greater customer loyalty .
Here’s what makes AI sentiment analysis stand out:
- Real-time processing: Get immediate insights from various channels.
- Multi-language support: Break down language barriers effortlessly.
- Consistent scoring: Standardized sentiment evaluation across all data.
- Pattern recognition: Spot trends and potential problems early.
What to Look for in AI Sentiment Analysis Tools
The right AI tools can help businesses efficiently process feedback and uncover actionable insights. For example, SentiSum's platform shows how AI can analyze data from multiple sources, providing detailed feedback that drives better decision-making .
Here are the must-have features:
- Multi-channel integration: Analyze feedback from social media, emails, surveys, and more - all in one place.
- Advanced language processing: Understand sarcasm, emotional undertones, and context for more accurate results.
- Customizable sentiment categories: Move beyond basic positive/negative labels to fit your business needs.
How to Integrate AI Tools for Sentiment Analysis
1. Initial Setup and Configuration
Begin with a pilot program. Set up the AI tool to focus on specific feedback channels and train it to recognize industry-specific terms and common customer phrases.
2. Data Connection and Validation
Connect all feedback sources, ensuring the data is clean and properly formatted. This ensures the tool delivers accurate results.
3. Performance Monitoring and Optimization
Keep an eye on the tool’s performance. Monitor key metrics like sentiment accuracy and refine the system using human validation to improve its effectiveness.
Choose tools that meet your current needs while leaving room for expansion. Pairing AI insights with visual data representations can make your findings even more actionable - a topic we’ll delve into next.
Displaying Qualitative Data
AI-driven sentiment analysis is just the beginning. To truly harness customer feedback, turning it into clear, actionable visuals is key for making smarter, faster decisions.
Methods for Data Visualization
Visual tools like clustering and sentiment comparison charts make it easier to spot trends and understand customer feedback. Here are three effective approaches:
- Theme Clustering: Groups similar topics to uncover common themes in feedback.
- Sentiment Comparison: Highlights positive vs. negative feedback across categories.
- Trend Mapping: Tracks sentiment shifts over time to detect potential issues.
"Data visualization makes customer feedback analysis simpler and quicker, saving your product team tons of time." - Userpilot Blog
Tools for Visualizing Feedback
Specialized tools help transform raw feedback into meaningful visuals through interactive dashboards. Here's a quick comparison:
Tool | Strengths | Use Case |
---|---|---|
MonkeyLearn | AI-powered sentiment analysis, topic grouping | Large-scale feedback analysis |
FreeText AI | Customizable reports, dynamic analytics | In-depth trend tracking |
Painboard | Real-time updates, interactive dashboards | Managing visual feedback quickly |
Using Visuals for Decisions
Visualizing feedback makes it easier to spot patterns that might go unnoticed in text. For example, UserPilot used these techniques to identify recurring customer issues, which helped them improve their product experience .
Good visualizations allow teams to:
- Pinpoint recurring issues with trend mapping.
- Combine feedback from multiple sources.
- Share insights effectively across departments.
To get the most out of your visuals, ensure data quality and accessibility for all stakeholders. This approach not only simplifies decision-making but also makes feedback instantly actionable - something we'll dive deeper into when discussing tailored feedback management systems.
sbb-itb-bca9945
Tailoring Feedback Management Workflows
Managing feedback at scale requires automated processes to turn raw data into actionable insights.
Improving Feedback Collection
Using multiple channels to gather feedback helps capture customer sentiment from various touchpoints. By integrating input from surveys, social media, support tickets, and direct interactions, organizations can create a unified feedback system.
Channel Type | Purpose | Key Benefits |
---|---|---|
In-app Surveys | Context-specific feedback | Real-time insights during product use |
Support Tickets | Detailed problem descriptions | Direct issue tracking and resolution |
Social Media | Brand sentiment and trends | Broader market perception |
Email Feedback | Detailed customer experiences | In-depth qualitative insights |
Automating Feedback Handling
Automation plays a critical role in turning raw feedback into meaningful insights. AI tools can streamline processes like categorization, sentiment analysis, and routing.
Key automation features to consider:
- Smart Categorization: Automatically sorts feedback by topic and urgency.
- Sentiment Detection: Flags negative feedback for immediate attention.
- Priority Assignment: Routes feedback based on risk and importance to the right teams.
Integrating Feedback into Operations
AI-driven analysis and visualization tools make it easier to incorporate feedback into daily operations. To ensure feedback leads to real improvements, maintaining high data quality is essential.
Steps to enhance operational integration:
- Embed feedback insights into product development workflows.
- Automate reporting across teams for better collaboration.
- Set up clear escalation protocols for critical issues.
- Regularly schedule reviews to track improvements.
These strategies lay the groundwork for addressing the data quality challenges discussed in the next section.
Ensuring Data Quality
Reliable data is the backbone of effective feedback analysis, especially as the volume of feedback grows. By streamlining processes and focusing on quality, you can ensure insights remain dependable over time.
Data Governance Practices
Managing feedback data effectively starts with clear governance. This involves setting up systems to control access, track changes, and comply with regulations. Here are the key components:
Component | Focus Area |
---|---|
Access Control | Role-based permissions and authentication |
Audit Trails | Automated logging and version management |
Compliance | Regular updates and monitoring of policies |
Data Security | Encryption and secure storage mechanisms |
Data Validation and Cleaning
AI-powered tools make it easier to check and clean data. Here's how they help:
- Duplicate Detection: Automatically spot and remove repeated entries.
- Completeness Check: Flag incomplete or missing information for review.
- Relevance Filtering: Remove irrelevant or spam submissions.
- Format Standardization: Maintain a consistent structure across all feedback channels.
These steps ensure your data is accurate and ready for analysis.
Maintaining Consistent Categories
Keeping feedback organized into clear categories is key for meaningful analysis. AI tools can assist by sorting feedback into predefined groups and adjusting to new trends.
Steps to maintain consistency include:
- Building a detailed classification system for feedback.
- Using AI to handle automatic categorization.
- Regularly reviewing and updating category definitions.
- Training your team to follow the classification guidelines.
Conclusion
Main Points
Using AI-powered tools helps businesses analyze growing amounts of feedback more effectively, leading to better customer experiences. Success depends on blending advanced technology with well-structured processes that can handle scaling demands.
Predictive analytics has become a game-changer, uncovering insights that manual methods might miss. By pairing AI with sentiment analysis, businesses are gaining powerful tools to understand customer feedback on a deeper level.
These advancements are paving the way for new technologies that will continue to reshape how feedback is analyzed.
Future Trends
As AI progresses, several developments are set to reshape feedback analysis:
- Anticipating customer needs through predictive systems
- Sentiment analysis with greater context awareness
- Integrating insights across multiple channels
The next wave of feedback analysis will focus on smarter, automated systems capable of managing large data sets and delivering actionable insights. Companies that adopt these technologies while ensuring strong governance will be better equipped to turn customer feedback into a competitive edge.