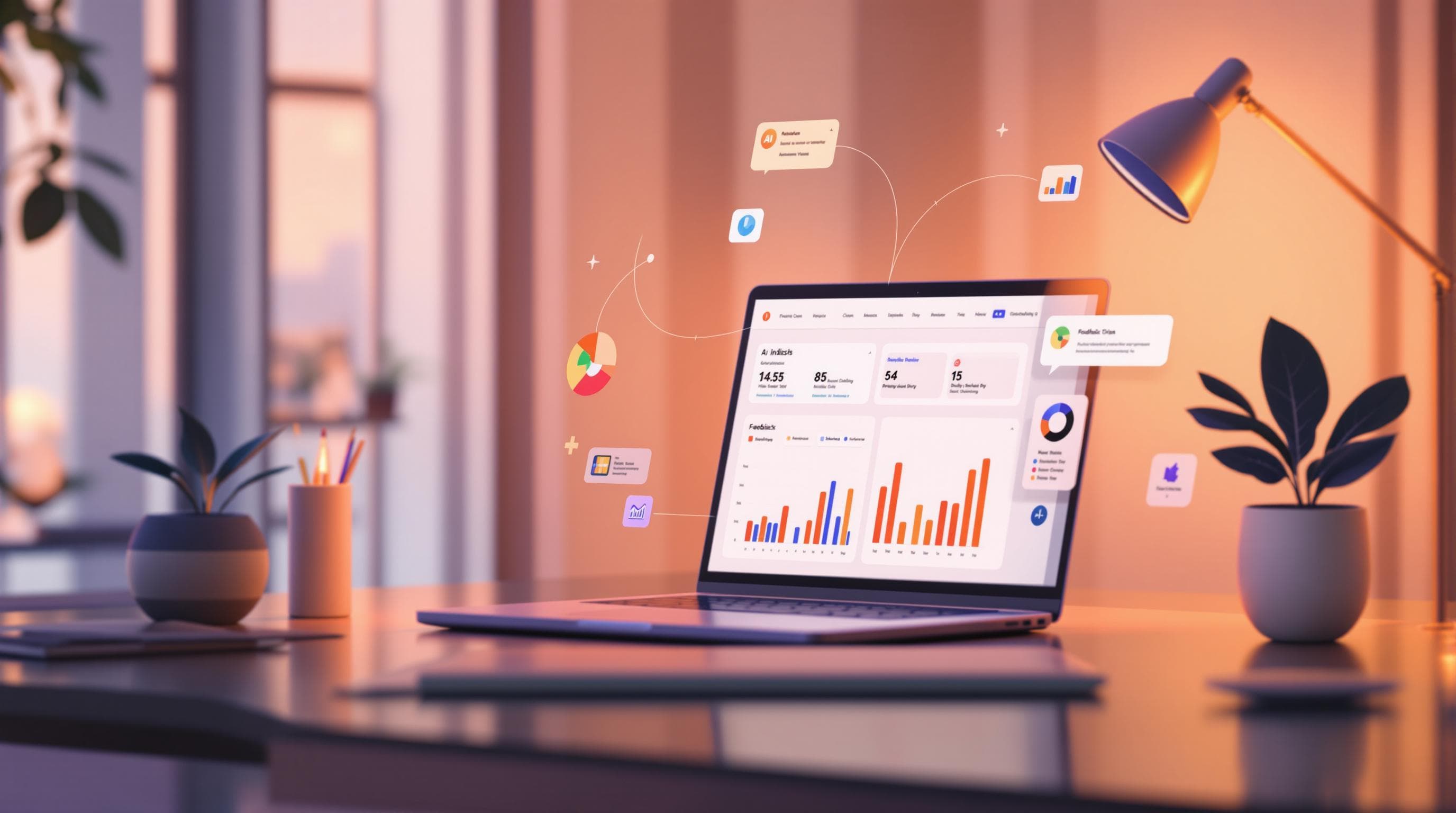
Scaling Feedback Analysis with AI: Best Practices
AI-powered feedback analysis helps businesses process large volumes of customer feedback quickly and accurately. It organizes feedback into themes, detects patterns, and delivers actionable insights. Here’s what you need to know:
- Why It Matters: Customer feedback shapes products, improves services, and drives decisions, but manual analysis is slow and inefficient.
- Key Benefits:
- Automated theme detection and trend identification
- Scalable processing for high data volumes
- Real-time insights with tools like Painboard
- Best Practices:
- Centralize feedback from all sources (e.g., surveys, reviews, tickets)
- Customize AI tools to fit your business needs
- Ensure data security with encryption and compliance
- Balance AI automation with human review for accuracy
- Choosing Tools: Look for features like sentiment analysis, theme grouping, and flexible dashboards. Match the tool to your data scale - basic, advanced, or enterprise solutions.
Quick Start: Centralize feedback, choose the right AI tool, and define success metrics (e.g., sentiment trends, resolution time). Use insights to make data-driven decisions across teams.
Setting Up Your Feedback Data System
Combining Feedback Sources
Bring together customer support tickets, survey responses, reviews, and call transcripts into one centralized system. This setup ensures all feedback is in one place, making it easier to analyze and act on. Key sources to include are:
- Customer support tickets
- NPS survey responses
- Product reviews
- Call center transcripts
By centralizing these inputs, your system can analyze different feedback types at the same time, paving the way for accurate AI-driven insights.
Data Quality Standards
To get reliable results from AI analysis, you need high-quality data. Consistency and reliability across feedback channels are critical. Here’s how to maintain high standards:
- Standardized Format: Ensure all feedback data is converted into a uniform format before analysis.
- Automated Validation: Use tools to catch incomplete or duplicate entries automatically.
- Context Preservation: Keep metadata like timestamps and source details intact.
- Theme Organization: Build a structured taxonomy tailored to your organization’s needs.
These steps ensure your data remains clear and actionable. Once your data is unified and checked, secure it using strong protocols.
Data Security Requirements
-
Data Protection
Protect sensitive information with automatic removal of personal identifiers, encryption, regular audits, and role-based access controls. -
Compliance Management
Align your feedback system with:- Data privacy laws
- Industry-specific regulations
- Internal security policies
-
Access Control
Implement a tiered access system to:- Restrict data visibility based on user roles
- Monitor user activity
- Keep detailed audit logs
These measures balance security with accessibility, ensuring your team can access customer insights without compromising data safety.
Selecting AI Feedback Analysis Tools
Features to Look For in an AI Tool
If you're scaling feedback analysis, your AI tool must manage large amounts of unstructured data and deliver actionable insights. Here's what to prioritize:
-
Advanced Analysis
- Perform sentiment analysis across multiple feedback channels.
- Automatically identify and group emerging themes.
- Spot patterns that indicate new trends.
- Convert qualitative feedback into measurable data points.
-
Customization Options
- Provide an adjustable taxonomy to align with your organization’s structure.
- Include personalized dashboards and tailored theme hierarchies.
- Allow flexible data segmentation to support specific workflows.
-
Technical Capabilities
- Efficiently process large-scale unstructured feedback.
- Ensure secure data handling, including removing sensitive information.
- Offer API access for easy integration with other tools.
- Support real-time data processing.
Comparing AI Feedback Platforms
AI feedback tools typically fall into three categories, each catering to different needs and scales:
- Basic Platforms: Ideal for organizations with lower feedback volumes. They usually provide simple AI-assisted theme detection, limited customization, basic integration options, and standard security.
- Advanced Platforms: Suitable for moderate data volumes, these tools feature more refined AI for detecting themes, moderate customization, and broader integration capabilities.
- Enterprise Solutions: Built for high-volume environments, these platforms offer extensive customization, advanced AI models, enterprise-level integrations, and robust security measures.
Selecting the right platform is just the beginning - you'll also need to ensure it integrates smoothly into your existing systems and scales effectively as your needs grow.
Integration and Scalability Considerations
Once you've identified the right platform type, focus on how it fits into your current workflows. Key factors include:
-
Data Flow Management
- Gather feedback from multiple sources into one centralized location.
- Automatically assign incoming data to the correct themes.
- Keep updates and synchronization in real time.
-
Scalability
- Adjust dynamically to improve categorization accuracy.
- Maintain consistent performance as data volumes grow.
- Learn from user interactions to enhance automated categorization.
-
Collaboration Features
- Offer shareable dashboards and reports.
- Provide team-specific views for focused analysis.
- Enable quick sharing of insights across departments.
Choose a tool that aligns with your organization’s workflow and can handle increasing feedback volumes. The system should continuously learn and apply past categorization decisions to new data automatically.
Creating Your AI Feedback Analysis Plan
Defining Success Metrics
Once you've set up your data system and selected the right tools, it's time to define how you'll measure success. Use clear metrics based on these performance indicators:
Metric Category | Key Performance Indicators | Measurement Method |
---|---|---|
Customer Experience | • Theme frequency trends • Sentiment changes • Issue resolution time |
Track changes across channels and periods |
Business Impact | • Revenue impact of issues • Customer retention rates • Implementation costs |
Compare performance across regions and market segments |
Operational Efficiency | • Analysis speed • Pattern detection rate • Theme accuracy |
Monitor the ratio of automated processing to manual review |
Creating Feedback Categories
Once your data is ready, organize feedback into clear, actionable themes. Create a taxonomy that’s structured but flexible enough to adapt to changing trends and business goals.
- Flexible: Your framework should evolve with new patterns. AI tools can identify emerging themes while keeping the structure aligned with your business needs.
- Structured: Use nested sub-categories to analyze both broad trends and specific issues. For example, a "product feedback" category could include sub-categories like features, usability, and performance.
- Context-Specific: Customize categories to reflect your industry and customer base. AI systems can learn from these adjustments and apply them to new data automatically.
AI and Human Review Balance
Combining AI with human oversight ensures both speed and accuracy. Start by using AI to handle large volumes of feedback - categorizing responses, spotting trends, and flagging items for human review.
Human reviewers step in for tasks like validating new themes, reviewing sensitive or high-priority feedback, refining category structures, and confirming AI-generated insights for critical decisions. When adjustments are made, they automatically update the system, improving future analyses.
Platforms like Painboard show how AI can integrate human input seamlessly, updating taxonomies and ensuring your insights stay relevant as customer needs evolve.
Striking this balance allows for fast processing while maintaining contextually accurate results.
sbb-itb-bca9945
Review customer feedback faster with AI summaries
Using Feedback Data for Business Decisions
Building on the earlier discussion about AI-driven feedback organization, this section dives into how businesses can turn those insights into actionable strategies.
Advanced Analysis Methods
Here are some key methods to analyze feedback effectively:
Analysis Type | Purpose | Key Benefits |
---|---|---|
Theme Taxonomy | Organize feedback into themes | Helps structure insights for quick use |
Cross-Channel Analysis | Compare feedback from multiple sources | Reveals patterns across touchpoints |
Trend Detection | Monitor sentiment and topic shifts | Identifies potential issues early |
Segmentation Analysis | Focus on specific customer groups | Highlights unique needs of each group |
These methods ensure feedback is not just collected but also structured in a way that can guide decisions.
Team-Specific Data Views
Different teams require tailored insights from the same pool of data:
- Executive Teams: Need high-level trends and shifts in customer sentiment.
- Product & Service Teams: Focus on hierarchical themes and popular customer topics.
- Customer Support Teams: Require detailed quotes and patterns of recurring issues.
Customizing the data view ensures each team gets exactly what they need to act effectively.
Converting Insights to Actions
Once feedback is analyzed and tailored for teams, it’s time to turn those insights into real-world strategies:
-
Data Organization
Organize over 970,000 pieces of feedback into themes for a complete picture of customer sentiment. -
Customization
Adjust themes and data views to align with your company’s specific goals and workflows. -
Monitor and Iterate
Use dynamic dashboards to track the impact of implemented changes. These dashboards can auto-route fresh feedback into the appropriate categories, keeping the process efficient and up-to-date.
Conclusion: Next Steps with AI Feedback Analysis
Key Points to Remember
AI-driven feedback analysis is changing how businesses handle customer feedback. To make the most of this technology, focus on these core components:
Component | Key Focus Areas | Benefits |
---|---|---|
Data Centralization | Gather feedback from all channels | Gain a complete picture of customer sentiment |
Customization | Align themes with your business structure | Generate insights tailored to your needs |
Security | Use PII removal and encryption | Keep customer data safe |
Scalability | Handle large feedback volumes | Perform thorough, large-scale analysis |
Use these principles to kick off your AI feedback analysis program with confidence.
Steps to Begin
Starting with AI feedback analysis works best when approached step by step.
Phase 1: Laying the Foundation
Start by bringing all feedback sources into one platform. This step highlights the value of centralizing data. At the same time, ensure security measures like encryption and PII removal are in place.
Phase 2: Fine-Tuning the System
Adjust the system to align with your business needs. This includes tweaking themes, creating dashboards for different teams, and refining analysis methods.
Optimization Tips
- Start small with a pilot program focusing on one feedback channel.
- Gradually include more data sources as you scale.
- Customize themes to match your business structure.
- Set up dashboards tailored to specific teams.
- Regularly review and tweak analysis settings to improve accuracy.